This example shows how to construct and conduct inference on a state space model using particle filtering algorithms. nimble
currently has versions of the bootstrap filter, the auxiliary particle filter, the ensemble Kalman filter, and the Liu and West filter implemented. Additionally, particle MCMC samplers are available and can be specified for both univariate and multivariate parameters.
Model Creation
## load the nimble library and set seedlibrary('nimble')set.seed(1) ## define the model stateSpaceCode <- nimbleCode({ a ~ dunif(-0.9999, 0.9999) b ~ dnorm(0, sd = 1000) sigPN ~ dunif(1e-04, 1) sigOE ~ dunif(1e-04, 1) x[1] ~ dnorm(b/(1 - a), sd = sigPN/sqrt((1-a*a))) y[1] ~ dt(mu = x[1], sigma = sigOE, df = 5) for (i in 2:t) { x[i] ~ dnorm(a * x[i - 1] + b, sd = sigPN) y[i] ~ dt(mu = x[i], sigma = sigOE, df = 5) } }) ## define data, constants, and initial values data <- list( y = c(0.213, 1.025, 0.314, 0.521, 0.895, 1.74, 0.078, 0.474, 0.656, 0.802) ) constants <- list( t = 10 ) inits <- list( a = 0, b = .5, sigPN = .1, sigOE = .05 ) ## build the model stateSpaceModel <- nimbleModel(stateSpaceCode, data = data, constants = constants, inits = inits, check = FALSE)
Construct and run a bootstrap filter
We next construct a bootstrap filter to conduct inference on the latent states of our state space model. Note that the bootstrap filter, along with the auxiliary particle filter and the ensemble Kalman filter, treat the top-level parameters a, b, sigPN
, and sigOE
as fixed. Therefore, the bootstrap filter below will proceed as though a = 0, b = .5, sigPN = .1
, and sigOE = .05
, which are the initial values that were assigned to the top-level parameters.
The bootstrap filter takes as arguments the name of the model and the name of the latent state variable within the model. The filter can also take a control list that can be used to fine-tune the algorithm’s configuration.
## build bootstrap filter and compile model and filterbootstrapFilter <- buildBootstrapFilter(stateSpaceModel, nodes = 'x') compiledList <- compileNimble(stateSpaceModel, bootstrapFilter)
## run compiled filter with 10,000 particles. ## note that the bootstrap filter returns an estimate of the log-likelihood of the model.compiledList$bootstrapFilter$run(10000)
## [1] -28.13009
Particle filtering algorithms in nimble
store weighted samples of the filtering distribution of the latent states in the mvSamples
modelValues object. Equally weighted samples are stored in the mvEWSamples
object. By default, nimble
only stores samples from the final time point.
## extract equally weighted posterior samples of x[10] and create a histogramposteriorSamples <- as.matrix(compiledList$bootstrapFilter$mvEWSamples) hist(posteriorSamples)
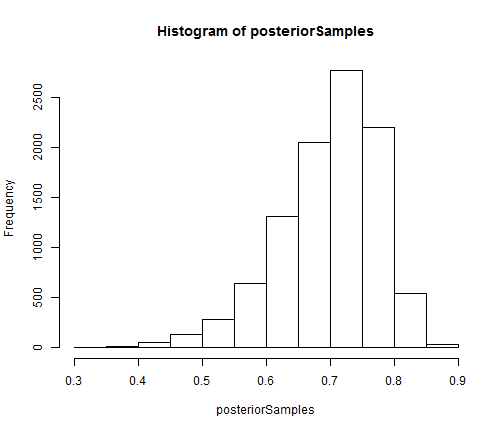
The auxiliary particle filter and ensemble Kalman filter can be constructed and run in the same manner as the bootstrap filter.
Conduct inference on top-level parameters using particle MCMC
Particle MCMC can be used to conduct inference on the posterior distribution of both the latent states and any top-level parameters of interest in a state space model. The particle marginal Metropolis-Hastings sampler can be specified to jointly sample the a, b, sigPN
, and sigOE
top level parameters within nimble
‘s MCMC framework as follows:
## create MCMC specification for the state space modelstateSpaceMCMCconf <- configureMCMC(stateSpaceModel, nodes = NULL) ## add a block pMCMC sampler for a, b, sigPN, and sigOE stateSpaceMCMCconf$addSampler(target = c('a', 'b', 'sigPN', 'sigOE'), type = 'RW_PF_block', control = list(latents = 'x')) ## build and compile pMCMC sampler stateSpaceMCMC <- buildMCMC(stateSpaceMCMCconf) compiledList <- compileNimble(stateSpaceModel, stateSpaceMCMC, resetFunctions = TRUE)
## run compiled sampler for 5000 iterationscompiledList$stateSpaceMCMC$run(5000)
## |-------------|-------------|-------------|-------------|## |-------------------------------------------------------|
## NULL
## create trace plots for each parameterlibrary('coda')
par(mfrow = c(2,2)) posteriorSamps <- as.mcmc(as.matrix(compiledList$stateSpaceMCMC$mvSamples)) traceplot(posteriorSamps[,'a'], ylab = 'a') traceplot(posteriorSamps[,'b'], ylab = 'b') traceplot(posteriorSamps[,'sigPN'], ylab = 'sigPN') traceplot(posteriorSamps[,'sigOE'], ylab = 'sigOE')
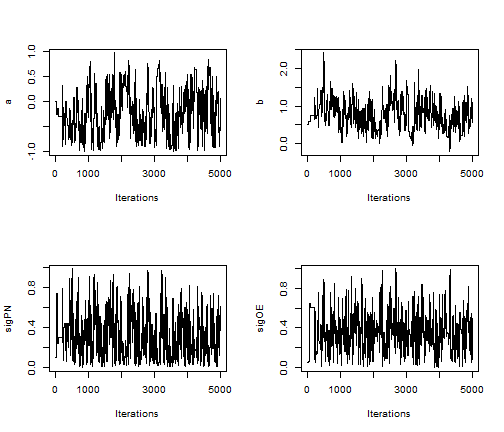
The above RW_PF_block
sampler uses a multivariate normal proposal distribution to sample vectors of top-level parameters. To sample a scalar top-level parameter, use the RW_PF
sampler instead.
转自:https://r-nimble.org/building-particle-filters-and-particle-mcmc-in-nimble-2